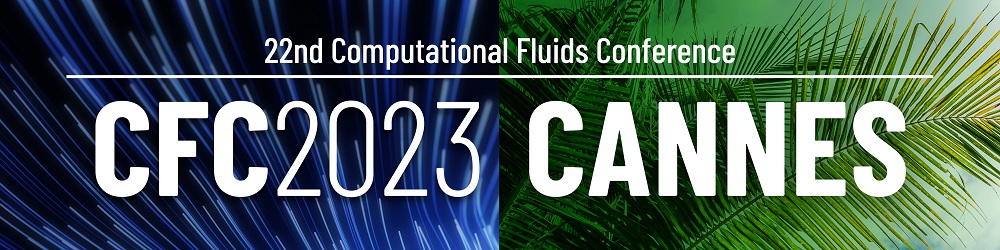
Deep Learning for the Computational Simulation of Pollutant Transport: an Error Model Perspective
Please login to view abstract download link
Detecting at early times the presence of pollutants in an aquifer is vital to take measures for mitigating their negative impacts. Identifying sources and understanding the spread of contaminants is critical in such a context. Those are particularly difficult missions due to the use of a small number of observation spots, which leads to computer models capable of conciliating monitored data with the final goals through the use of inversion formulations. The efficacy of inverse problems strongly relies on an adopted forward model’s predictive ability and computational efficiency. Indeed, both aspects are commonly contradictory, as simplifications of the modeling are assumed to alleviate costly computations. Here, we investigate the impact of employing phenomenological state equations to characterize the sorption of pollutants in a physics-based model that combines first principles with closure relations (transport mechanisms). We phrase it as a probabilistic error model and employ a Deep Learning surrogate to enable the analysis.