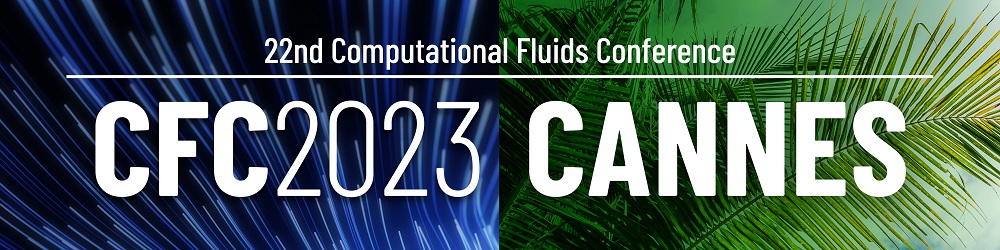
Efficient Multi-Fidelity Estimation of Boundary Conditions for Cardiovascular Hemodynamics
Please login to view abstract download link
Patient-specific computational models of the vasculature require calibration of boundary conditions (BCs) to account for the patient’s physiology. BC calibration is an inverse identification process that iteratively evaluates the computer model until predefined quantities of interest from the computational model output match with reference values from literature or clinical measurements. However, the solution of inverse problems with high-fidelity 3D cardiovascular models is difficult to achieve due to the prohibitively large computational cost. 0D lumped-parameter networks, on the other hand, can quickly predict bulk flow and pressure values with just slightly reduced accuracy. Thus, 0D models are instrumental in BC calibration, where many repeated evaluations are required. The computational speed-up of the 0D models allows us to account for uncertainties in the calibration problem by performing Bayesian inverse analysis. We propose a new multi-fidelity framework that shifts the workload of parameter estimation to 0D models while accurately capturing the posterior distribution from the 3D model through repeated 3D-0D updates. Parameter estimation is performed entirely on 0D models, and only a few 3D evaluations are used to validate the estimates and update the 0D model. The Bayesian framework employs Sequential Monte Carlo (SMC) using 0D models to derive even multimodal posterior distributions for unknown BC parameters, resulting in robust estimates and accurate uncertainty quantification. We show how the repeated 3D-0D updates effectively increase the correlation between the 0D and 3D model. Moreover, the identifiability of the Bayesian inverse problem is analyzed. We further show that the 3D-0D multi-fidelity strategy significantly reduces the computational cost for parameter estimation (only a handful of 3D evaluations are required) and can be applied to a wide range of model anatomies (aortic, pulmonary, and aortofemoral) from the Vascular Model Repository (https: //www.vascularmodel.com).