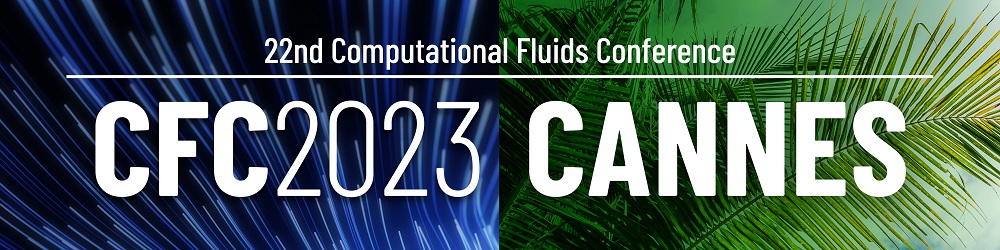
Predicting near-optimal meshes for CFD simulations
Please login to view abstract download link
The process of designing modern complex engineering components requires the use of computational simulations. It is essential for the computational simulations to determine consistent solutions accurately, achieved by using meshes that have been refined to ensure they capture the solution appropriately. Ensuring the appropriate refinement of the meshes often requires extensive human interaction by experienced engineers, using either mesh adaptivity or manually refining the mesh spacing function based on the observed solution. In this talk, we propose methods to minimise the human interaction required using artificial intelligence (AI) techniques to capture the knowledge embedded in previous mesh convergence studies. The two techniques proposed for describing the optimal spacing function for training the AI are based on the two most common uses of mesh control. The first method for describing the optimal spacing function uses point sources. For a given solution, the technique determines the optimal spacing function that captures all the features of the solution and subsequently produces a set of sources. Furthermore, the technique then combines all the sources from various parameters into a single set of global sources, which can then be used for training the neural network that can predict the characteristics of the sources for a given parameter [1]. The second method for describing the optimal spacing function uses a background mesh. For a given solution the method determines its corresponding optimal spacing function and interpolates the spacing function onto a reference background mesh [2]. As part of the interpolation process, a conservative approach to interpolating is taken to ensure the new interpolated spacing function does not under-refine the optimal mesh. Additionally, as part of the interpolation process for varying geometry, a technique that maintains the background mesh topology is devised. The two schemes are applied to a variety of three-dimensional compressible flow cases to demonstrate the potential of the various proposed approaches. The examples will include: the varying of flight conditions and geometry.