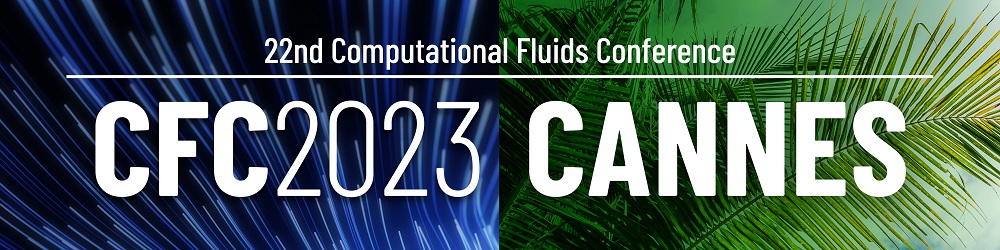
Mesh-based graph neural networks surrogates for mechanics problems with changing domains
Please login to view abstract download link
We propose a neural network (NN) based surrogate for the approximation of elastic and hyper-elastic stationary mechanics problems trained with mesh-based ground-truth simulations. Our surrogate is an extension of MeshGraphNets [1] to time-independent mechanics problems. The latter are particularly challenging because they require fast propagation of the information throughout the whole domain. We improve MeshGraphNets using two techniques: 1) by augmenting the baseline network with nonlocal connections, or 2) by training the network hierarchically, in a multi-grid manner. Both approaches have the effect of enabling the immediate propagation of information between points in the domain that are far apart. We test the proposed surrogate on two-dimensional problems characterized by changing domains, materials, and boundary conditions. Our results illustrate the generalization properties of the surrogate, which can accurately predict unseen scenarios.