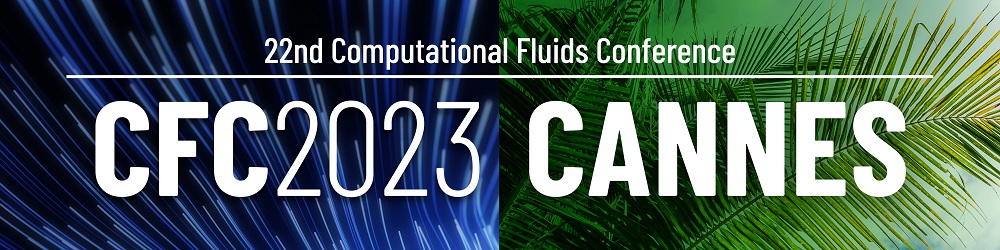
Explainable transfer learning for stable and generalizable data-driven LES
Please login to view abstract download link
In this work, we develop a data-driven subgrid-scale (SGS) model for large eddy simulation (LES) of 2D turbulent thermal convection using a fully convolutional neural network (CNN). In the big-data regime or with data augmentation, the LES-CNN reproduces accurate statistics in terms of kinetic energy and entropy (scalar energy) spectra and Nusselt number. Although LES-CNN works when the training and online testing systems have the same control parameters, e.g., Rayleigh number and Prandtl number, the network suffers from inaccurate inferences when it is applied to a different flow with different control parameters. We propose to use transfer learning to address the generalization/extrapolation issue. Transfer learning requires a small fraction of the big data obtained from the target system and a trained network with big data obtained from the base system. To understand the physics of transfer learning, we explain what is learned in transfer learning to a different turbulent flow, which is based on changes in the convolution kernels of the base network after re-training to the target network and the kernels’ physical interpretation. We show a general a priori framework to guide transfer learning of similar systems.