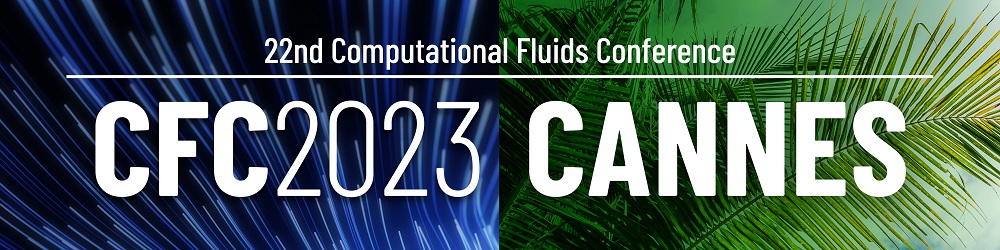
Networks-based characterization of arterial flow spatiotemporal complexity
Please login to view abstract download link
The definition of “coherent structures” in cardiovascular flows is elusive, generally meaning regions of characteristic organization, recurrence, appreciable length scale and lifetime during the cardiac cycle. Notwithstanding the frequent use of the term, there is no method able to clearly identify and isolate these structures. To fill this gap, the description of coherent flow structures given by Robinson [1] as structures over which one fundamental macroscopic quantity exhibits significant correlation with itself over a significant scale of space and/or time represents a useful starting point. Building on this description, we applied the Complex Networks (CNs) theory to in silico and in vivo hemodynamic data [2,3] to uncover the organization and spatiotemporal similarity of large-scale arterial flow structures. Based on graph theory, a CN is defined by a set of nodes and a set of links connecting the nodes. Each node is defined as the grid point of the finite element computational mesh used to generate in silico hemodynamic data or as the voxel of in vivo 4D flow MRI acquisitions. A link between two nodes i and j is established if the Pearson correlation coefficient R_ij between time-histories of the hemodynamic quantity under investigation (e.g., velocity magnitude) at those nodes is greater than a threshold value R ̂. Such threshold value can be based on statistical grounds (e.g., median value) or on physical considerations (e.g., zero, to discriminate positive and negative correlations). The information on nodes connection is used to build a so-called connectivity matrix, which is then used to extract quantitative parameters assessing, e.g., the degree of dynamical similarity of blood flow structures, or the distance over which a hemodynamic quantity persists along the vessel length. The application of CNs to several arterial districts in normal and pathological conditions allows to pin down in which way specific anatomical attributes disrupts flow coherence (e.g., in the carotid bulb [2] or in dilated ascending aortas [3]), and to underscore the effect of disturbed flow features like flow separation, jets, or highly rotational flow on global flow organization. The introduced paradigm provides a quantitative definition of coherent hemodynamic structures, improving the characterization of complex blood flow features and potentially accelerating their clinical translation in risk stratification and classification criteria.