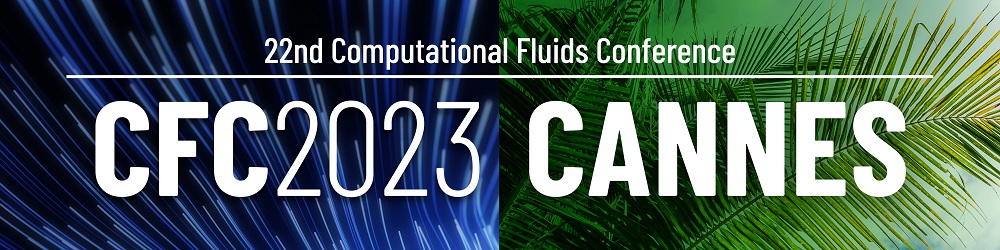
Gaussian Process Emulators as Surrogate Models for Four Chamber Cardiac Hemodynamics
Please login to view abstract download link
Simulating the total heart function includes computational fluid dynamics (CFD) blood flow models which serve as the hydrodynamic load imposed on cardiac mechanics. Heart valves play a pivotal role in filling and ejection of cardiac chambers. Thus, their functional representation in CFD simulations of hemodynamics in the human heart and the attached vessels is vital. Usually this task is achieved using fluid-structure-interaction (FSI) where valves are modeled as thin structures. We investigate the suitability of an alternative approach: A fictitious domain method is realized by extending the Navier-Stokes equation with a linear permeability term, which results in the Navier-Stokes-Brinkman equations. In this setting the permeability parameter is used to model a valve as a fictitious solid domain. The (fast) opening and closing of the valve is realized by changing the permeability within the finite elements which are covered by the moving valve in its current configuration. The underlying mesh representing the blood pool remains unchanged but the equations contain a volume fraction parameter denoting the degree of partial coverage of finite elements in the blood pool by the valve. To deal with turbulence occurring at higher Reynolds numbers the residual based variational multiscale (RBVMS) turbulence model was employed. The RBVMS formulation has the additional property of stabilizing our method, which allowed the use of lowest equal order finite elements reducing also the implementation work in the cardiac modeling framework CARPentry. In this talk we will present ongoing validation work, sensitivity analysis using probabilistic surrogate models and applications stemming from clinical datasets.