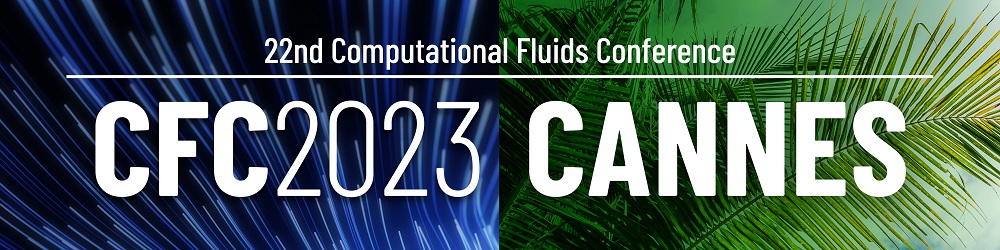
Machine Learning Augmented Turbulence Modelling for Massively Separated Three-Dimensional Flows
Please login to view abstract download link
Accurate simulations of turbulent flows are critical to engineering design in many industries, such as aerospace, automotive, architecture, wind energy, and nuclear energy. The dominant method to simulate turbulent flows is Reynolds-Averaged Navier-Stokes (RANS) simulations, which have many well-known shortcomings. Machine learning offers a way to increase the accuracy of RANS, at relatively small computational costs. The primary source of error in a RANS simulation is often the turbulence model, which is required to model the unclosed term (the Reynolds stress tensor). Traditionally, these models are constructed from a physics-based standpoint, by introducing an eddy viscosity hypothesis and adding transport equations for other scale-determining variables such as the turbulent kinetic energy (TKE) and dissipation rate. These various approximations required to close the RANS equations produce a number of coefficients, which are usually tuned by hand. Hand-tuning limits these heuristic methods, which can only express simple or moderately complex closure relationships. On the other hand, data-driven approaches offer the ability to discover a richer and more sophisticated closure relationship. Recently, machine learning techniques to augment closure relationships in RANS simulations have resulted in several frameworks being proposed. However, effectively none of these methods have been demonstrated to scale up to large datasets, and the applicability of these methods for a complex 3D flow has not been sufficiently tested. In this work, we will demonstrate that machine learning can be used to augment RANS simulations of massively separated 3D flows, by using a large dataset containing over twenty million points. This dataset consists of several large eddy simulations (LES) and RANS simulations of separated turbulent flow over a series of obstacles. We will present key modifications to an existing closure framework, enabled through access to this large dataset. These include modifications that introduce critical properties such as invariance under translation, rotation, and Galilean transformation. After training and testing a machine learning model on this large dataset, we will demonstrate that the accuracy of machine learning augmented RANS simulations can approach the accuracy of LES simulations for this complex 3D flow.