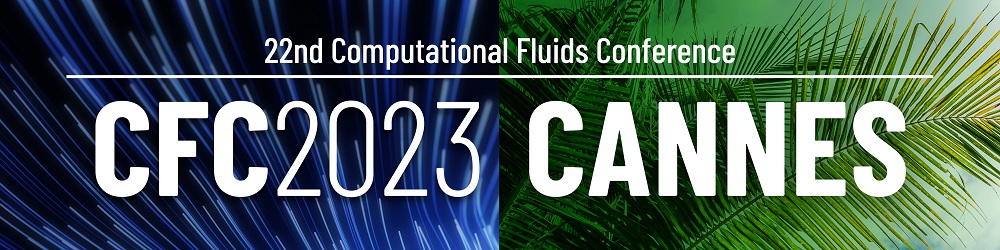
Facilitating atmospheric source inversion via deep operator network surrogates
Please login to view abstract download link
Climate effects are caused by a combination of confounding sources which interact with the climate system through various feedbacks. The identification of sources in climate systems, be they natural or a result of human intervention, is a vital problem for attribution and prediction of climate states. Due to the inability to isolate sources in nature and the computational cost of climate simulators, surrogate models are required to enable the many-query algorithms required for exploration of the inverse problem of source identification. We introduce a framework based on (1) calibrating deep operator network surrogates to the flow maps provided by an ensemble of atmospheric dispersion simulations obtained by sources with different parameters, then (2) using these surrogates in a Bayesian framework to identify sources from observations via optimization. The expressive and computationally efficient nature of the deep operator network surrogates allows for source identification with uncertainty quantification in complex system from comparatively limited data.