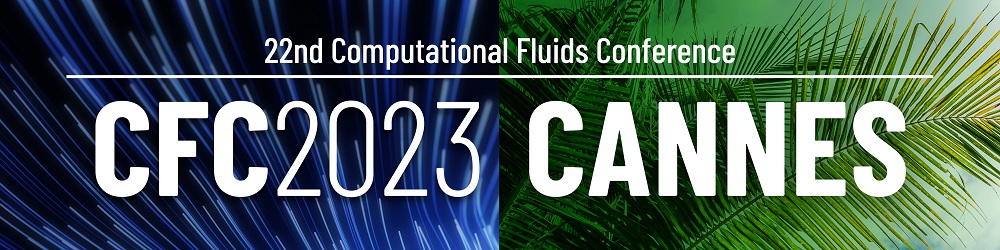
Embedded Learning of Closure Models for Filtered Discrete Equations
Please login to view abstract download link
Computing turbulent fluid flows on coarse discretizations typically involve the use of spatial filters to remove high-frequency components. These filters do not generally commute with the differential operators, and closure models are applied to obtain equations for the filtered variable only. For large eddy simulation (LES), these closure models are traditionally postulated in a continuous setting. In a previous work, we compared approaches to learn discrete closure models for the application of non-uniform filters to discretized linear equations. In this work, we extend this framework to non-linear equations. The discrete closure models are trained to reproduce reference trajectories for the filtered solution in time while embedded in the solver. The same models are also trained to predict the correct reference closure terms only. Recent works suggest that the latter approach may achieve high accuracy for the closure terms (sub-grid scale stresses) while still leading to instabilities once embedded in the solver. In contrast, the former approach trains the model to enforce stability, but the cost function has a significantly larger computational graph involving discrete time stepping. The length of the time trajectories may thus lead exploding or vanishing gradients. For the considered training approaches, we compare the accuracy, stability, computational cost of training, and generalization capacity to longer simulation times and new initial conditions. We also compare different regularization approaches, choices of hyper-parameters (such as number of time steps unrolled), and need for high-fidelity training data.