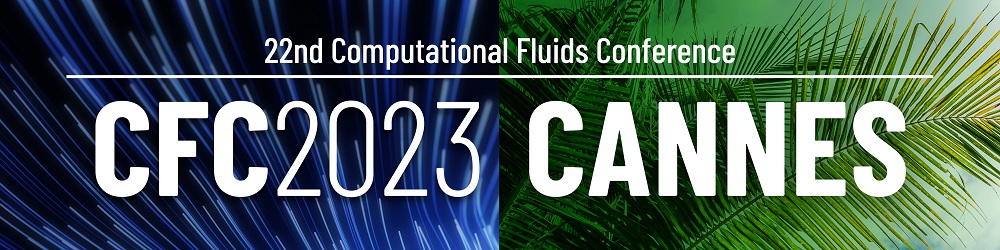
Assessment of a Data-Driven RANS Model for Various Separated Flows
Please login to view abstract download link
Accurate prediction of separated flow is essential in analyzing aerodynamics of flight vehicles. Reynolds-averaged Navier Stokes (RANS) approaches have been widely used in such aerodynamic simulations. However, it is well known that a typical RANS turbulence model is limited in predicting separated flow. In this study, the prediction capability of RANS model is improved using a machine learning approach. The field inversion and machine learning (FIML) method proposed in Parish and Duraisamy is applied to improve the Spalart-Allmaras (SA) model. Various separated flows are simulated for model improvement. Flow fields around an airfoil at high angles and separated flow around axisymmetric bodies are used to improve the SA model. The modified SA model is able to predict separated flows in new flow conditions with the variation of the Mach and Reynolds numbers for the same geometry. Moreover, the modified SA model is tested across various geometries. The variation of geometry includes both minor changes, such as nominal, flared, and boattail bases of an axisymmetric body, and a major geometry change between an axisymmetric base body and an airfoil. Application of the data-driven SA model on different geometries is assessed. Successful applications are noticed in the current study with both minor and major changes in geometry. Not-so-successful cases are also assessed, which can improve the model training process with expanded data sets. More details and related discussion will be provided in the presentation.