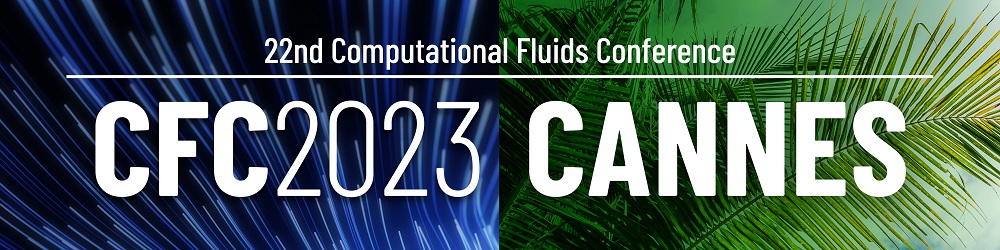
Machine Learning Method for Surrogate Substitution of Obstacles in CFD Simulations
Please login to view abstract download link
Complex flow problems involving small rigid body obstacles embedded in large computational domains lead to very large meshes and small time steps, impacting simulation performances. When only the highest scales information is relevant, a way to improve performance consists in substituting the complex geometries by ”models” of lower resolution. In the proposed implementation, complex high resolution obstacles are substituted with lower resolution zones of variable anisotropic porosity, included in the Navier-Stokes equations, which mimics the presence of obstacles. The porosity tensor is obtained numerically by a Machine Learning method for arbitrary obstacles and flows. The method has been tested on a variety of obstacle geometries for different flow conditions and the resulting flow characteristics have been compared in terms of velocities, pressure drops and momentum variations.