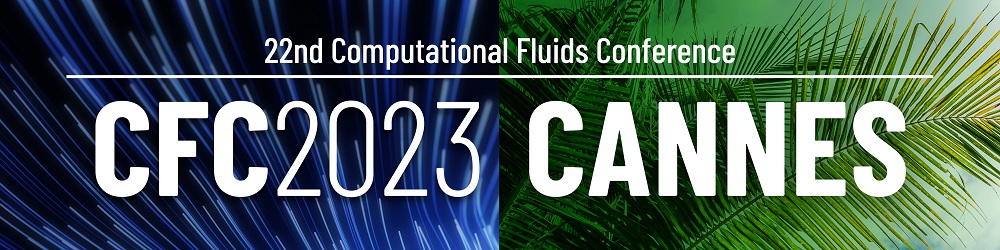
Leveraging Differentiable Physics to Efficiently Learn Flow Past Arbitrary Geometries
Please login to view abstract download link
The present paper builds on the ideas formulated by Um et al. [1] and presents an efficient hybrid framework capable of obtaining an accurate representation of fluid flows past arbitrarily shaped complex bodies. The challenge of accurate reconstruction of the fluid velocity field is further steepened by the presence of multiple bodies that cause abrupt local (i.e., within the vicinity of the geometry) changes in the velocity field while also exhibiting vortex shedding at multiple frequencies. Consequently, the focal point of training a model is shifted to accurate local (e.g., boundary layer profile) as well as global (e.g., vortex shedding frequencies) flow characteristics. The open-source immersed boundary-based continuum flow solver FoamExtend v4.0 is used as a reference solver to generate accurate training data. The hybrid framework is realized by coupling the in-house open-source differentiable simulation toolkit φFlow as the source solver with a continuous convolution-based neural network model. Such a coupling reaps the benefits of training feedback over long time horizons and has been shown to avoid the instability of supervised learning approaches. Suitable comparisons are also made with baselines comprising a supervised learning (SL) based approach and a reduced-order model-assisted supervised learning approach (ROM-SL). Finally, care has been taken to formulate a loss function that strikes a balance between near-boundary physics and wake dynamics. Results indicate that the present hybrid differentiable physics-assisted convolution neural network (DPCNN) framework out- performs the baselines. Moreover, the reconstructed flowfield is shown to closely obey latent dynamics.