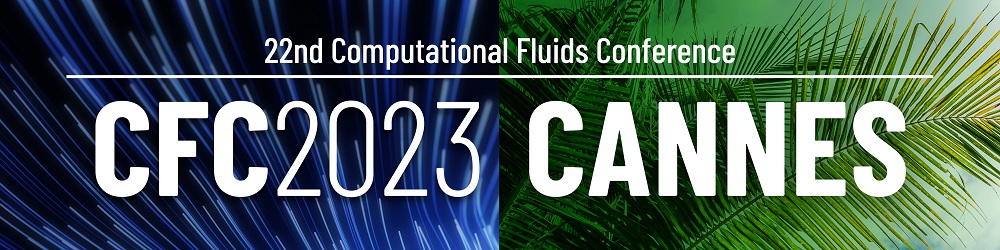
Reduced-Order Models of Magnetoconvection in 2D
Please login to view abstract download link
Direct numerical simulation of full state equations can be computationally intensive and result in high-dimensional datasets. This has led to an interest in finding reduced order models for such systems. To identify a reduced-order model, we make use of proper orthogonal decomposition. The time series of these modes projected on the measurements can then be used to generate a reduced order model via the sparse identification of nonlinear dynamics (SINDy) [1]. We apply this method to 2D magnetoconvection, where traditional convection can be inhibited by the presence of a magnetic field. These convective processes are important, for instance, at the surface of stars. It was shown that the governing equations for magnetoconvection admit weakly nonlinear solutions given by a system of 5 ODEs [2] valid close to onset. These are instructive as they are able to predict features such as whether convection first sets in as a direct instability or overstability. Further, numerical simulations cannot find unstable steady solutions, which can be found by this truncated model, allowing for a more complete picture of the phase space. Stability criteria can then be determined with much greater ease than by simulation of the full PDE system. However, these equations are only valid near the onset of instability, when the velocities are small. We first apply these methods to understand if recovery of the analytic solutions near fixed points is possible, before looking for reduced order models further from onset in regimes with aperiodic motion. By comparison with the solutions of the full PDEs, we assess the utility of the reduced models both for prediction and for reproducing the statistics of the full system; we further test how well a model derived for a certain set of parameters reproduces the dynamics for parameter sets for which no direct data is available.