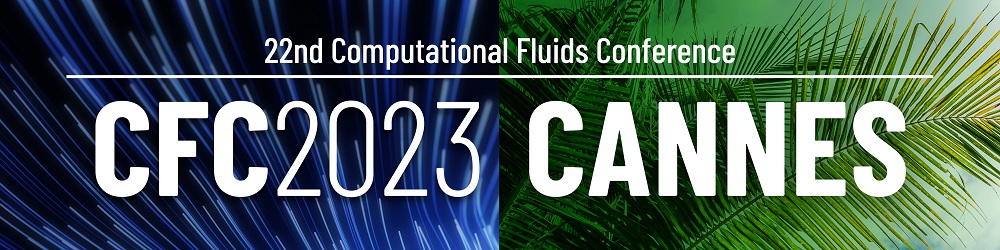
Structure-Preserving Machine Learning: Energy-Conserving Neural Network for Turbulence Closure-Modelling
Please login to view abstract download link
In turbulence modelling, and more particularly in the LES framework, we are concerned with finding a suitable closure model to represent the effect of the unresolved subgrid-scales on the larger/resolved scales. In recent years, the scientific computing community has started to gravitate towards machine learning techniques to attempt to solve this issue. However, stability and abidance by physical structure of the resulting closure models is still an open problem. To resolve this, we take the discretize first and filter next approach starting out from a high-resolution reference simulation. We apply a spatial averaging filter to reduce the degrees of freedom and derive a new kinetic energy conservation condition that takes into account both the resolved and unresolved scales. We then suggest a data-driven compression to represent the subgrid-scale content on the coarse grid in order to comply with our new conservation condition. Finally, a skew-symmetric convolutional neural network architecture is introduced that can be enhanced with dissipative terms to account for viscous flows. Combined with a structure-preserving discretization this framework is used to evolve both the filtered solution and the compressed subgrid-scale representation in time in a structure-preserving fashion, yielding non-linear stability, while still allowing for backscatter. We apply the methodology to both the viscous Burgers’ equation and Korteweg-De Vries equation in 1-D and show increased accuracy and stability as compared to a standard convolutional neural network. We also evaluate the performance of our approach for different levels of filtering and observe convergence to the reference solution as this level is reduced.