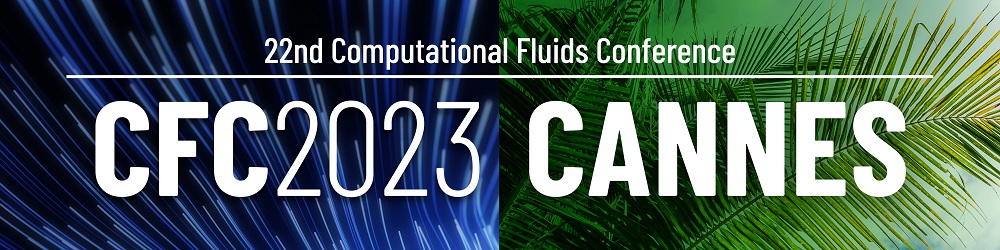
Uncertainty Quantification and Calibration of One-Dimensional Arterial Hemodynamics
Please login to view abstract download link
Cardiovascular diseases are the leading cause of death globally. Virtual twins can support healthcare professionals in the diagnosis, interventional planning, and follow-up of patients. Three-dimensional (3D) high-fidelity computational hemodynamics simulations are usually extremely costly. One-dimensional (1D) and zero-dimensional (0D) models enable lower-fidelity non-linear simulations of the cardiovascular system for a fraction of the 3D computational cost and with sufficient accuracy for medical purposes. To make these models patient-specific, they must be calibrated against clinical measurements of the patient's anatomical and physiological features. For lower fidelity 1D-0D models, the calibration amounts to learning hundreds of parameters from observations. Even if the computational cost of the 1D-0D model is low, solving the inverse problem for its parameters can be demanding. In this work, we investigate Neural Networks (NN) as surrogates of the 1D-0D models to accelerate the calibration. We propose different NN architectures, suggested by the physics of the blood flow and the mathematical structure of the model, to predict time signals of blood pressure, flow rate, and artery cross-sectional area. We train these NNs on a synthetic data set (solutions of the 1D-0D model) corresponding to a priori ranges of variation for the parameters, and we contrast their respective predictive accuracies. Then, we conduct sensitivity analyses and calibrate the parameters using the NNs. The best NNs have average prediction errors below 5% for our test problems, and "patient-specific" parameters are identified with similar error levels.