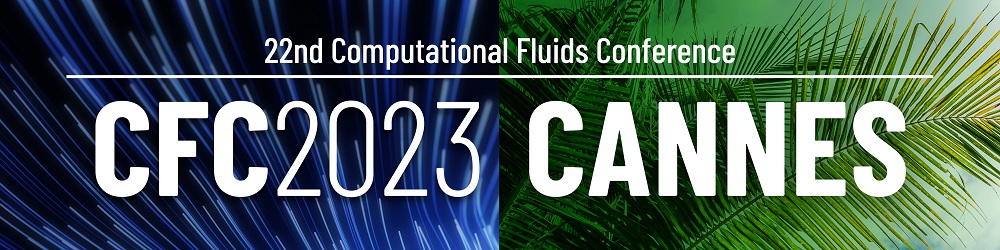
Reconstruction of Turbulent Flows Using Generative Diffusion Models
Please login to view abstract download link
Generative Modelling has shown significant promise in the field of subgrid-scale closure for turbulent flows [1, 2, 3]. State-of-the-art Generative Adversarial Networks (GANs) allow for high-fidelity reconstruction of turbulence from coarse representations which can then be used to provide subgrid closure. However, limitations of adversarial training have hindered the wider adoption of GANs in subgrid closure modelling. An unstable Nash equilibrium between generator and discriminator and mode collapse are often encountered in training [4]. These issues can be somewhat mitigated through regularisation and optimisation tricks, but architecture design and hyperparameter optimisation remain a careful balancing act between stability and computational expense. To circumvent the drawbacks of GANs, the aim of the present study is to investigate the use of Generative Diffusion Models (GDMs) in super-resolution enhancement of coarse-grid turbulent flow fields, with a view towards restoring missing physics in the form of subgrid closure terms. GDMs are a new development in the field of Generative Modelling. In contrast to GANs, which use an adversary to implicitly learn the underlying statistics of a dataset, GDMs iteratively sample from the reverse stochastic differential equation (SDE) associated with a diffusion process used to corrupt the data via a Markov-chain of Gaussian transitions. Using approaches inspired by Langevin dynamics, at inference time the score is calculated and samples from the induced PDF are obtained iteratively [5]. With improvements in stability and conditioning, GDMs present a significant advantage over GANs. We investigate the recovery of missing physics in a stably stratified Kelvin-Helmholtz instability shear flow, with turbulent phenomena and body forces acting on the flow [6]. We use a super-resolution GDM to restore missing physics from unresolved Large Eddy Simulation (LES) data, by extracting subgrid-scale information from predicted high-resolution fields. The full presentation will include a rigorous examination of generated samples through the lens of turbulent flows: turbulence spectra, two-point correlations, higher order statistics, and scalar dissipation will be analysed.