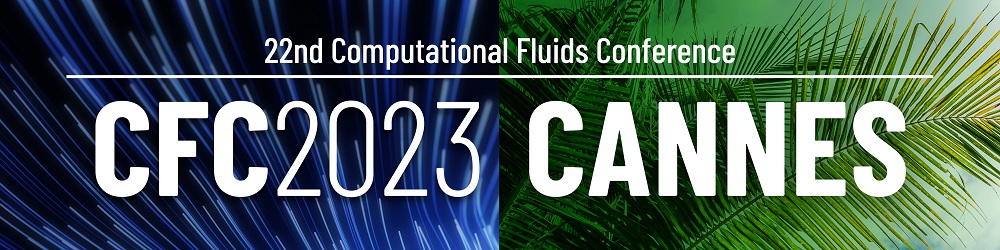
Data-enhanced personalized models for coronary hemodynamics and myocardial perfusion
Please login to view abstract download link
This work demonstrates a framework for more accurate and personalized coronary hemodynamics models that leverage novel non-invasive CT myocardial perfusion imaging (MPI-CT) and anatomical morphometric data. We create more physiological coronary artery models by combining incomplete image-based anatomies with synthetic vascular trees for non-imaged/under-resolved arteries. We generate synthetic vascular trees using Constrained Constructive Optimization based on published coronary morphometric data. The hemodynamics is simulated using the open-source SimVascular software suite. Multi-scale 3D and reduced-order models of flow are coupled with closed-loop lumped parameter models for distal systemic circulation. Furthermore, these models are tuned to match personalized coronary flow distributions derived from MPI-CT, as well as other clinical measurements of cardiac function, by using surrogate-based optimization to estimate model parameters. These flow simulations are coupled with finite-element models of myocardial perfusion based on Darcy flow. We compare these data-enhanced CFD models with traditional methods for simulating patient-specific coronary hemodynamics and myocardial perfusion, and compare with clinical MPI-CT.