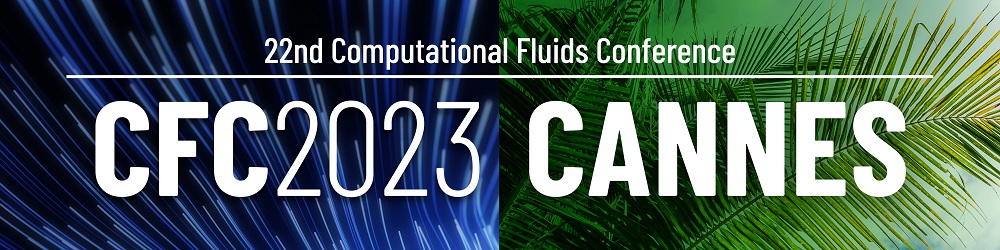
Latent space data assimilation with uncertainty quantification using deep learning
Please login to view abstract download link
Data assimilation is considered a crucial task in many modern industrial settings and is essential in digital twins. However, data assimilation remains a challenging task due to the often high-dimensionality and nonlinearity of high-fidelity models. This is especially the case when one is interested in distributional information about the state and parameters. In this case, one must compute or sample from a posterior distribution over all degrees of freedom. In this talk, we present a non-intrusive methodology that speeds up Bayesian data assimilation by utilising a particle filter approach and replacing the computational bottlenecks with neural network-based surrogate models. The high-dimensionality is dealt with using adversarial autoencoders to reduce the high-dimensional high-fidelity state to a low-dimensional latent state. Thereafter, a transformer is used for time advancement in the latent space. Embedding the surrogate models into a particle filter, enables data assimilation in the latent space which results in a latent space posterior distribution. This ensures highly efficient state and parameter estimation with uncertainty quantification. The proposed methodology is tested on the important engineering problem of leak detection in pipelines. This problem is modeled by a set of nonlinear hyperbolic PDEs with a discontinuous source term, which makes it difficult to solve for conventional methods in a satisfying time frame.