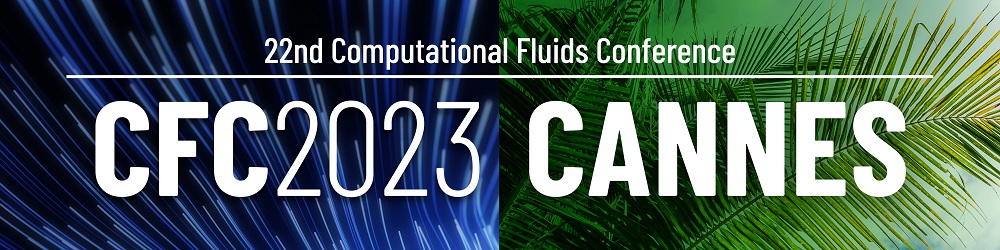
Automatic Mesh Optimization and Machine Learning: A Path to Enabling A Wider Use of Controlled-Accuracy 3D CFD in Industry
Please login to view abstract download link
In RANS-based CFD, meshing remains a time-consuming part of any 3D simulation. Automatic mesh generation remains a holy grail of CFD, with each situation still requiring considerable human intervention before resulting in an acceptable grid. No matter how many tools are introduced to facilitate mesh generation around complex geometries, the fact remains that CFD has “singular features” such as boundary layers, shock waves, vortices, shear layers, and separated flows that render the Navier-Stokes equations ill-conditioned, besides being coupled and highly nonlinear. These singular phenomena cannot be identified nor localized before launching a solution and hence no grid could claim to be able to, without knowing where the locations of the particular physics it is trying to resolve will turn out to be, capture all such features with great fidelity. The “systematic refinements” to reach a mesh-independent solution advocated by some CFD standards committees and required by some journals will be shown to be akin to “looking where the light is instead of where the keys are”. These approaches may, at great cost, gradually lead to a better solution but by no means to an optimal one. The present paper will strive to demonstrate that the most logical, and perhaps only, way of building efficient grids ought to be by coupling mesh generator and solver.