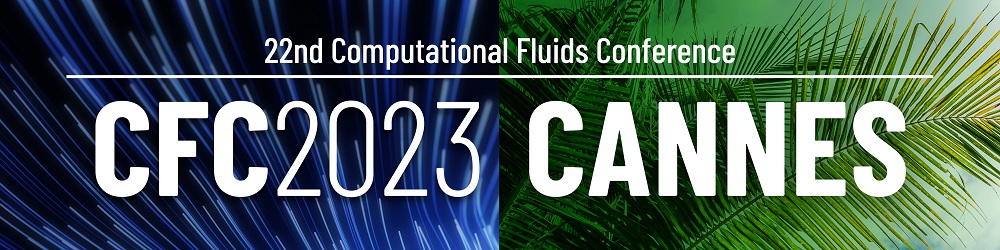
Artificial Neural Networks Based Closure Models for Reduced Order Models in Computational Fluid Mechanics
Please login to view abstract download link
The truncation of projection based Reduced Order Models (ROMs) can result in stability and inaccuracy issues. A two-step closure modeling approach is presented in this work to address these two issues. This work uses an a priori approach, focused on providing the required stabilization, followed by an a posteriori approach to achieve the desired accuracy for the ROMs. In the first step, a Variational Multiscale (VMS) concept based stabilization using Orthogonal Subgrid Scales is employed to stabilize the ROMs. In the second step, an Artificial Neural Networks (ANNs) based correction term is added to the fully discrete system, i.e. discretized in both space and time, to improve the accuracy of the ROMs. Considerations regarding the data generation for training ANNs are also discussed in detail. The implicit and explicit treatment of ANN-based correction term in the nonlinear iterative scheme is also evaluated. The performance of the resulting corrected ROM is evaluated for the reconstruction and temporal-extrapolation phases for the flow around a cylinder and the flow over a backward facing step. It is also shown that the ANN-based correction can recreate the non-periodic flow around triple cylinders in a triangular formation using only 20 modes, whereas, ROM without the ANN-based correction fails to do so even with 80 modes. The corrected ROM is also shown to produce good results for the parametric interpolation and extrapolation cases for the flow over a cylinder with Reynolds number (Re) being the parameter of interest. Finally, the corrected ROM is shown to produce good results even when both, time and Re, are extrapolated beyond the training interval.