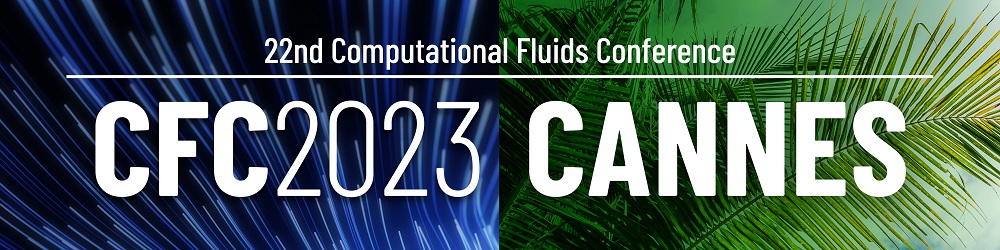
Active flow control on 3D cylinders through deep reinforcement learning
Please login to view abstract download link
he interest in aerodynamic performance improvements increases rapidly, and there is a need to apply it massively to the aeronautical industry and others transport involving a high resource consumption. Flow control together with data-driven methods can provide more efficient strategies. Deep artificial neural networks (DANNs) used with reinforcement learning (RL), also called Deep reinforcement learning (DRL), are getting popular to explore and manage complex systems in many areas. In this particular work, an ANN trained through a DRL agent, coupled with the numerical solver Alya, is used to perform active flow control (AFC). The Tensorforce library, from Tensorflow, is used to apply DRL to the simulation. The learning has been applied to discover new AFC strategies that can minimize the drag of the obstacle, in this case, a 2D cylinder. The control is possible thanks to two jets located at the surface of the cylinder. The agent, through a proximal-policy optimization (PPO), knows how to interact optimally with these jets changing the mass flow rate. In this contribution, a cylinder in a three-dimensional domain will be considered at low Reynolds where the agent will have to deal with more complex structures and the computational cost will optimized through multi-environment approach. There are successful results with the 2D cylinder at low and high Reynolds [1] [2] [3], seeing how the control is able to modify the wake, increasing the recirculation bubble and reducing the drag by 8% at Re = 100 and approximately 17% for Re = 2000.