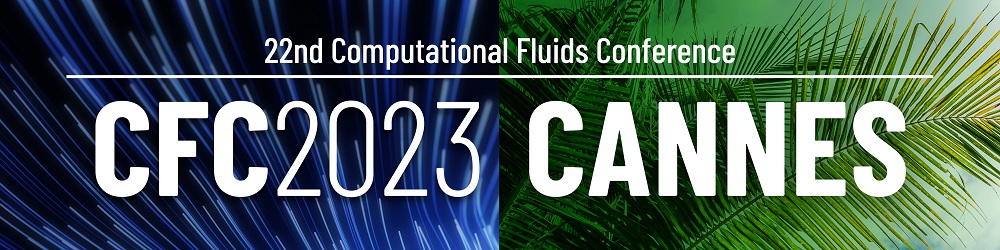
Learning models from single and multiple sources for fluid problems in engineering design and optimization
Please login to view abstract download link
This talk will focus on computational strategies that build upon the synergies across machine learning and scientific computing to overcome the limitations that affect traditional data-intensive and domain-agnostic learning approaches. In particular we will provide and overview of methods that we developed to learn domain-informed models from single and multiple representations of the fluid flow to support design and optimization problems in engineering. Different engineering applications will be considered to illustrate the methods and solicit a discussion about open challenges and opportunities.