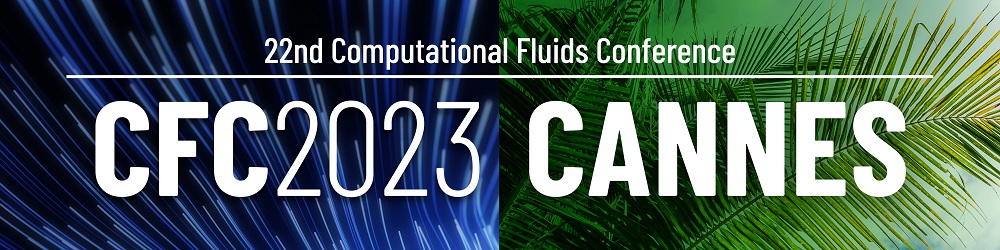
Towards Real Time Bayesian Inversion and Prediction of Megathrust Tsunamis
Please login to view abstract download link
Tsunamis generated from megathrust earthquakes are capable of massive destruction. Efforts are proceeding to instrument subduction zones with seafloor acoustic pressure sensors to provide early warning for tsunamis. Our goal is to employ this pressure data, along with the coupled acoustic--gravity wave equations, to infer the spatiotemporal seafloor deformation in a real time early warning system. The solution of this inverse problem then provides the boundary forcing to forward propagate the tsunamis toward populated areas along coastlines. Solution of the forward problem alone---the acoustic--gravity wave equations in a 3D compressible ocean---entails severe computational costs stemming from the need to resolve ocean acoustic waves with wavelength of order 150 meters. The inverse problem formally requires thousands of forward wave propagations, and accounting for uncertainties in the inversion and predictions is several orders of magnitude even more costly. Thus our goal appears to be intractable. We propose a novel approach to enable the accurate solution of this pair of inverse and prediction problems in real time, i.e., in seconds. We discuss the Bayesian formulation, consistent discretization, and fast solvers, and provide numerical illustrations for model geometries.