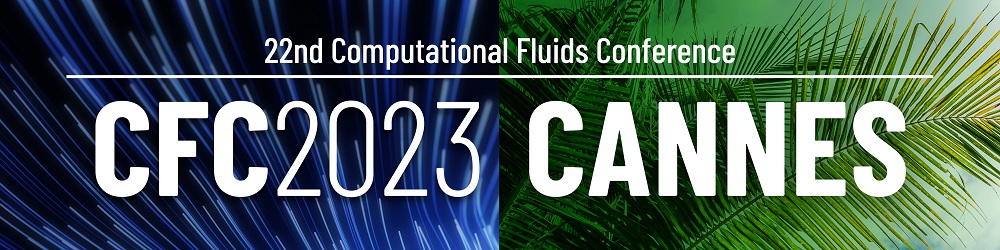
Keynote
Differentiable programming for Hessian-based ocean climate observing system design in the North Atlantic
Please login to view abstract download link
We illustrate derivative-based, Hessian uncertainty quantification and its underlying concepts in the context of designing an effective observing system in the subpolar North Atlantic that maximally informs the target QoIs. The approach is made possible through rigorous use of reverse-mode automatic differentiation to generate the adjoint a state-of-the art ocean circulation model, akin to the use of backpropagation in machine learning.