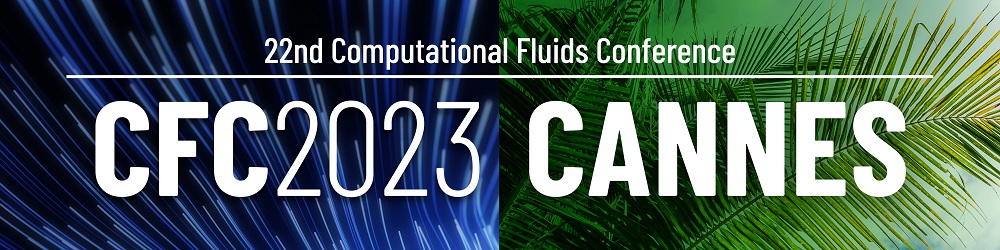
Strategies for improving the performance of Physics-Informed Neural Networks for predicting flow fields in bioreactors
Please login to view abstract download link
Fermentation and cell cultivation in bioreactors are essential tools in biotechnological research and production. Due to the lack of suitable measurement techniques, little information on the production processes and environmental conditions of the cells is available. Computational Fluid Dynamics (CFD) can be used to simulate flow fields in bioreactors and enable the prediction of velocity and pressure, as well as specific characteristics, such as mixing times. However, high-fidelity simulations are computationally intensive, especially in optimization and control scenarios, where the same model must be solved multiple times for different parameter values. This motivates the construction of Reduced Order Models (ROMs) to approximate high-fidelity solutions at lower computational cost. In this work, we are investigating the applicability of Physics-Informed Neural Networks (PINNs), originally proposed by Raissi et al. [1] as ROMs for stirred tank bioreactors. PINNs are a machine learning concept well suited for engineering problems, where data is typically sparse and costly to obtain and the governing equations are known. By embedding the governing equations into the loss function of the neural network, the amount of data needed to train the network is decreased significantly. The use case is particularly challenging, not only because of the large variety of phenomena involved in a fermentation process (e.g., turbulence, mass transfer), but also due to the geometric complexity of the computational domain. The predictive accuracy and training time of the model can be improved by leveraging additional knowledge, such as symmetry of the problem or domain decomposition based on different character of the flow in different parts of the domain. We also aim to investigate methods that improve the training process itself, such as locally adaptive activation functions or dynamic weighting of the loss function components. Strategies to improve the overall performance of the model that can be applied to other complex problems will be presented.