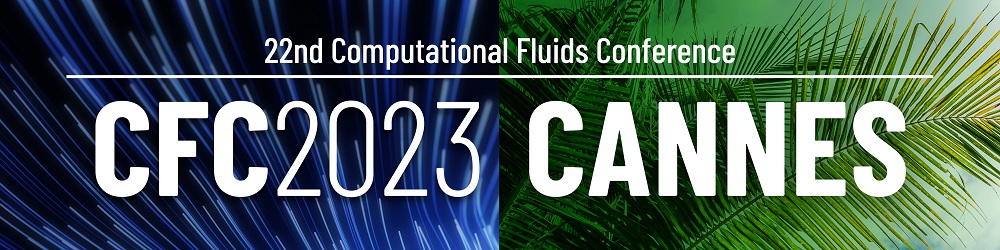
Nonlinear reduced-order modelling from data
Please login to view abstract download link
A reduced-order modelling of very large, nonlinear systems remains a major challenge despite advances in computational power. Projection-based model reduction techniques have been in use for this purpose but they rely on ad hoc mode selection and produce a priori unknown errors. In this talk, I discuss a recent mathematical alternative to these approaches based on spectral submanifolds (SSMs), which are very low dimensional, attracting invariant surfaces in nonlinear systems. Reduction to SSMs turns out to yield previously unimaginable speed-ups in solving large finite-element models. Very recent results also show that SSMs and their reduced dynamics can be constructed directly from data. I will illustrate these results on numerical and experimental flow data sets.