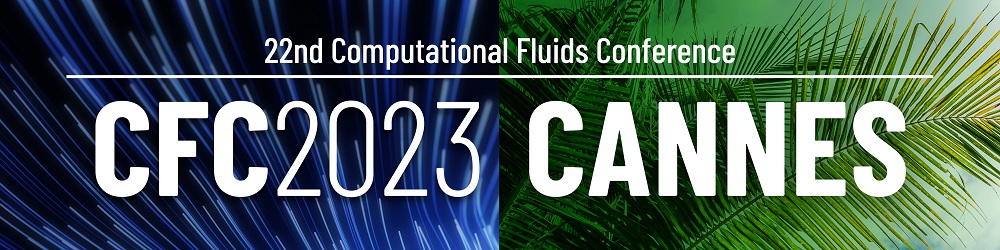
The Deep Learning Inference of Compressible Flows Over an Aerofoil
Please login to view abstract download link
Recent years have witnessed great advances in the applications of deep learning to fluid mechanics. Owing to its significance in both fundamental research and industrial practice, the present study investigates the accurate inference of Reynolds-averaged Navier-Stokes (RANS) solutions for the two-dimensional compressible flow over aerofoils with a deep neural network. In most existing studies, a constant, uniformly distributed auxiliary grid is used to infer RANS solutions with deep learning methods. The auxiliary grid itself takes the form of a Cartesian grid, and hence the geometric input G takes the form of a binary mask or a signed distance function to encode the embedded aerofoil. However, an inherent drawback is that the discretization of the physical domain for inference can not change. As a consequence, the discretization has to be redundantly fine to avoid the undesirable blurring of important flow structures. To overcome those challenges, we propose an alternative approach where the domain discretization varies and adapts to each input. Information about this discretization and aerofoil coordinates is encoded in G. The proposed method's accuracy, robustness, and performance are analyzed for new shapes and free-stream conditions that never appear in the training set. In a benchmark case of incompressible flow at randomly given angles of attack and Reynolds numbers, we achieve an improvement of more than an order of magnitude compared to previous work. Further, for transonic flow cases, the deep neural network model accurately predicts complex flow behavior at high Reynolds numbers, such as shock wave/boundary layer interaction, and quantitative distributions like pressure coefficient, skin friction coefficient as well as wake total pressure profiles downstream of aerofoils. The proposed deep learning method, being orders of magnitude faster than the conventional CFD solver, yields significant computational speedups, and shows promise for enabling fast aerodynamic designs. As the model is differentiable, it can be seamlessly integrated into gradient-based optimization algorithms. Moreover, being trained with varying mesh deformations, the neural network architecture is able to deal with changing geometries and can be easily extended to boundary value problems of PDEs discretized with structured body-fitted grids.