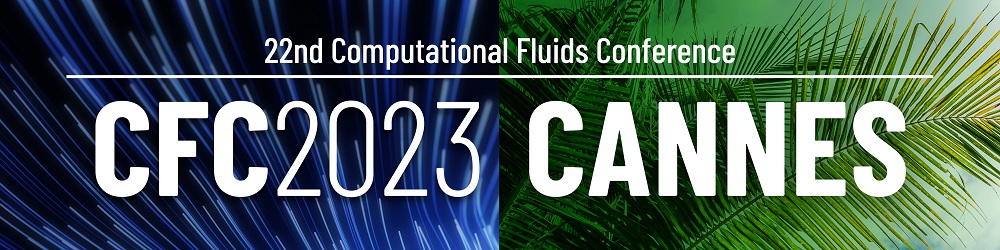
Improvements for Uncertainty Estimation in Active Learning – Towards Automated Multifidelity Metamodels
Please login to view abstract download link
Multifidelity methods have become widely used in simulation-based design optimization, to model the behavior of the problem. They combine the effective exploration ability of low-fidelity simulations with a few accurate high-fidelity simulations to produce more precise predictions. The performance of a metamodel is dependent on an adequate and reliable sampling methodology, so a well-defined adaptive sampling strategy may profoundly accelerate the training and improve the accuracy of metamodels [1]. It is vital to use an adaptive sampling strategy that is based on reliable uncertainty estimation, to obtain accurate predictions from the metamodel. Any deficiency in the uncertainty estimation may result in either inadequate exploration of the design space, or oversampling in unnecessary regions. Therefore, the metamodel may become too expensive to train or it may even dismiss the global optimum and get stuck in a trivial region. Finally, uncertainty can be used as a decision tool for fidelity level selection which underlines the fact that efficient uncertainty estimation is a crucial part of the metamodeling processes. In the present study, we propose two contributions to improve the uncertainty estimation for multifidelity surrogate models. The first one is adjusting the uncertainty estimation in regions where only few data are available, since our existing estimation may not be reliable unless a certain number of data points is provided for the metamodel. Therefore, we implemented adjustment criteria to tune the uncertainty based on the data point density in the neighbourhood of the predicted location. Moreover, for noisy simulation cases, it is challenging for the metamodel to reach an acceptable level of accuracy, since the data points may not be reliable because of the associated noise. Therefore, the second contribution aims to divide the uncertainty estimation into its components as data-point and interpolation uncertainty, then estimate these separately which will provide a more reliable multifidelity metamodel. The performance of the modified uncertainty estimation will be assessed and compared for a selected set of analytical benchmark problems. Then, the proposed improvements are applied to the shape optimization problem for a two-dimensional NACA four-digit airfoil, based on CFD simulations. 1 J. Wackers, R. Pellegrini, M. Diez, A. Serani, M. Visonneau. Improving active learning in multi-fidelity hydrodynamic optimization. 34th SNH, 2022.