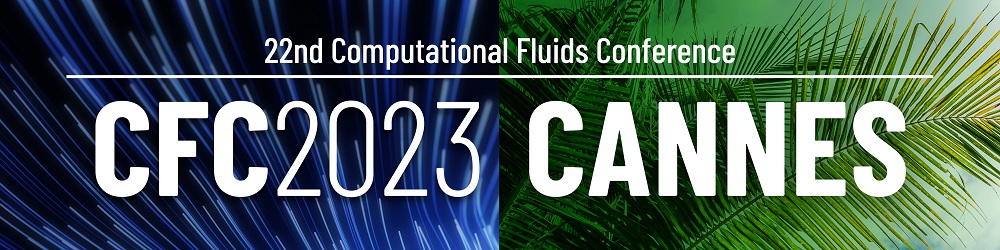
CNNs and autoencoders for surrogate models of a rocket engine injector from Large Eddy Simulations
Please login to view abstract download link
The design of chemical rocket propulsion systems is under growing pressure of reducing development costs. The use of CFD codes for the simulation of combustion chambers can provide an economical alternative to costly experiments which have traditionally been at the core of liquid rocket engine (LREs) developments. Nonetheless, a holistic approach for design analysis and optimization is not yet practical, as the exploration of entire engine design space via high-fidelity numerical simulations is too expensive. Appropriate surrogate models may circumvent this dilemma through fast inference times, without significant accuracy loss. In the present work, the focus has been laid in the liquid rocket engine injector. The injectors are a key element within LREs as they are responsible for introducing and mixing the propellants into the combustion chamber. Large Eddy Simulations (LES) of multiple shear-coaxial injector configurations, operating with a gaseous oxygen-methane mixture, have been carried out with the AVBP solver. The conceived design-space spans across three parameters, two geometrical (the oxidizer post recess and chamber radius), and one operational (the mixture ratio). From this dataset, U-Nets and MLPs have been used for the elaboration of parametric surrogates of relevant 2D field quantities. Furthermore, autoencoders have been trained and used to analyze the different flow realizations in the latent-space, enabling the identification of distinct dynamics in the design-space, and the elaboration of time-evolving, parametric surrogates of the injector.