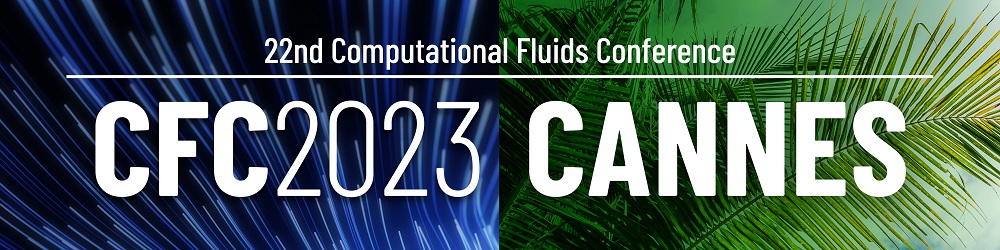
Subspace-Distance-Enabled Active Learning for Non-Intrusive Reduced-Order Modeling of Parametric Fluid Flows
Please login to view abstract download link
In scenarios where repeated evaluations for varying parameter configurations of a high-fidelity physical model are required, surrogate modeling techniques based on model order reduction are used. The reduced basis method [1, 2], equipped with a residual-based error estimator, is one possible methodological choice. There, snapshots at new parameter samples are generated and the reduced basis space is greedily updated via an iterative procedure. However, when the governing equations of the dynamics are not accessible, we need to construct the parametric reduced-order model (ROM) in a non-intrusive fashion. In this setting, the residual-based error estimate for optimal parameter sampling is not available. Our work intends to provide an alternative optimality criterion to efficiently populate the parameter snapshots, thereby, enabling us to efficiently construct a parametric non-intrusive ROM. We present an active learning approach to efficiently build a non-intrusive ROM by greedily picking important parameter samples from the parameter domain. As a result, during the offline ROM construction phase, the number of high-fidelity solutions dynamically grow in a principled fashion. The high-fidelity solutions are expressed in parameter-specific linear subspaces, and the relative distance between these subspaces is used as a guiding mechanism to perform active learning. To achieve this, two distance criteria are proposed in this work. The active learning procedure is initiated with high-accuracy snapshots for a preliminary coarse parameter set. We then sample new parameter locations by progressively subdividing the farthest subspaces, in an iterative fashion, based on the distance criteria. We also present heuristically sound strategies to perform this subdivision between any two selected subspaces. Additionally, a non-intrusive error estimator is used to ensure a user-specified accuracy level for the ROM solution. At the end, a non-intrusive parametric ROM based on kernel-based shallow neural networks is actively learned with the proposed framework. To demonstrate the validity of our ideas, we present numerical experiments using two parametric fluid flow models: viscous shallow water equations and the incompressible Navier-Stokes equations.