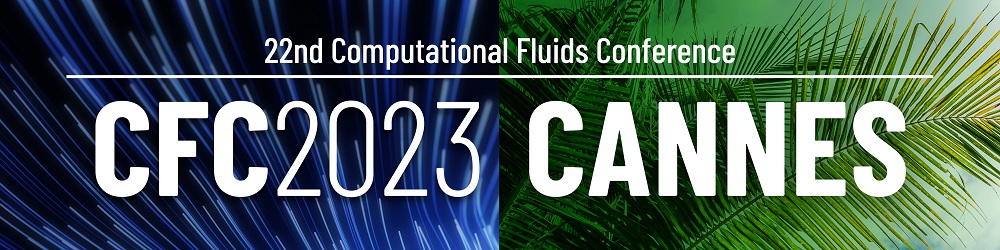
Efficient Genetic Programming for data-driven RANS model discovery
Please login to view abstract download link
We aim to derive RANS closure models based on high-resolution (LES) ground-truth data. Genetic programming (GP) can obtain parsimonious closures (simple expressions) that minimize RANS vs ground-truth misfit, but at the cost of a large number of function evaluations - each of which is a CFD solve. This high cost is the limiting factor to widespread application of this approach - to the extent that training on 3d flows is currently impractical. We demonstrate the basic technique, and accelerate the method with three improvements: 1. We use residuals of the candidate models with respect to the ground-truth as a fitness filter - eliminating unpromising models without a solve; 2. We adjust a modern evolutionary algorithm (Gene-pool optimal mixing EA), which builds a statistical model for the relationships between parts of the expression tree, allowing more effective mutation and recombination; 3. We use gradient-based constant optimization within the EA. Combined, these improvements give us a practical means of obtaining models customized for classes of 3d flows.