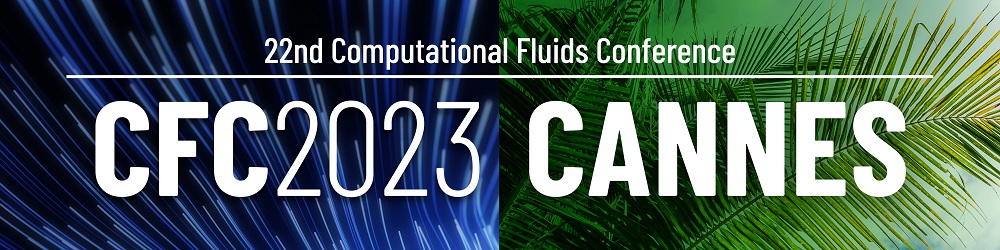
Prediction of Flow Characteristics of the Three-Dimensional Wavy Wing using CNN and Encoder-Decoder Models
Please login to view abstract download link
The wavy geometrci distrbance is one of the passive flow control to improve the aerodynamic performace of the airfoil. The present study considers the semi-span wing which is geometrically defined by the aspect ratio. In addition, the waviness is charaterized by the wavelength and the wave amplitude. Thus, these geometric parametres lead to the numerous conbinations which need very heavy case studies. Therefore, this study aims at establsihing the deep learning model to lessen the heavy loads of the experiments and alsonumerical simulations.The present study considers two different convolutional neural network (CNN) models to predict the three-dimensional wavy wing. The evaluation of the drag and lift as the aerodynamic performance of airfoils is essential. In addition, the analysis of the velocity and pressure fields is needed to support the physical mechanism of the force coefficients of the wavy wings. Thus, the present study aims at establishing two different deep learning models to predict force coefficients and flow fields of the airfoil. One is the CNN model to predict drag and lift coefficients of airfoil. Another is the Encoder-Decoder (ED) model to predict pressure distribution and velocity vector field. The images of airfoil section are applied as the input data of both models. Thus, the computational fluid dynamics (CFD) is adopted to form the dataset to training and test of both CNN models. The models are established by the convergence performance for the various hyperparameters. The prediction capability of the established CNN and ED modes is evaluated for the various NACA sections by comparing the true results obtained by the CFD, resulting in the high accurate prediction. It is noted that the predicted results near the leading edge, where the velocity has sharp gradient, reveal relatively lower accuracies. Therefore, the more and high resolved dataset are required to improve the highly nonlinear flow fields.