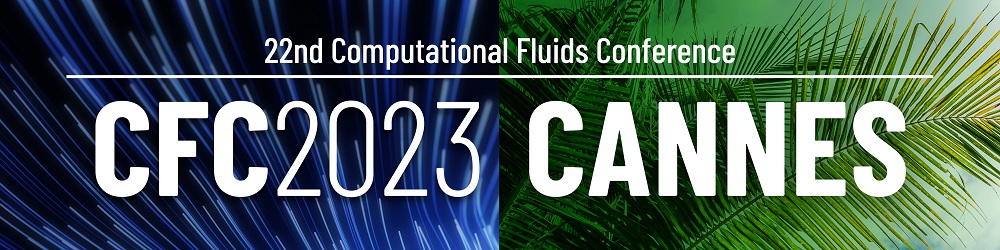
Dealing with faulty sensors: a physics-informed convolutional neural network approach for recovering solutions to governing equations
Please login to view abstract download link
Measurements on dynamical systems, experimental or otherwise, are often subjected to inaccuracies capable of introducing corruption. Removal of this is a problem of fundamental importance in the field of fluid dynamics, for instance in PIV measurements [1]. Research on correcting measurements predominantly considers noise-removal and flow-reconstruction [2]. In this work we propose a form of flow-reconstruction to extract the true, underlying solution to the Navier-Stokes equations from biased data with access to only limited ground-truth observations. We introduce a form of physics-informed convolutional neural network, embedding prior knowledge of the physics in the form of the governing equations [3] and exploiting spatial correlations. We showcase the methodology for three physical systems: linear convection-diffusion, non-linear convection diffusion, and the 2D turbulent Kolmogorov flow. Measurements are subjected to corruption, adding stationary, spatially-varying bias parameterised by frequency and magnitude; showing of the methodology to both frequency and magnitude of the corruption. By conditioning sparse ground-truth observations on the governing equations, we demonstrate a novel method to recover the underlying flow across the entire domain. This work opens opportunities for the extraction of Navier-Stokes solutions from PIV data and the detection of faulty sensors that introduce biases [4].