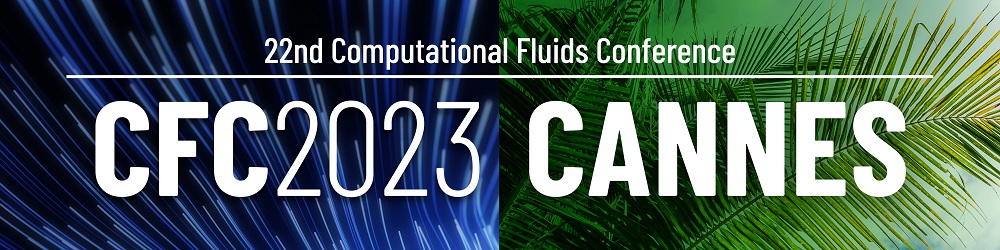
Enhancement of Subgrid-Scale Turbulence-Chemistry Interaction Physics-Based Models: A Data-Driven Perspective
Please login to view abstract download link
In Large Eddy Simulations (LES) of combustion, model deficiencies might result from simplified closure models. Traditional combustion models can lead to inconsistencies between model predictions and observations, particularly when employed to simulate non-conventional fuels and combustion regimes. That is the case of subgrid-scale (SGS) turbulence-chemistry interactions (TCI) closures for LES, wherein the filtered reaction rates can not be directly computed from the filtered thermo-physical quantities, due to non-linearity. Identifying and understanding possible deficiencies of such models is vital to take measures for mitigating their negative impact. Reactor-based models have attracted interest as TCI closures in LES since they can deal with finite-rate chemistry at an affordable computational expense. Here, we focus on the based-reactor models and their associated cell-reacting fraction sub-model. More specifically, we combine machine learning and sparsity-promoting techniques to improve the predictive capabilities of based-reactor models by modeling new functional forms of the cell-reacting fraction sub-model from data-driven techniques. Therefore, the obtained functional forms are parsimonious models that balance goodness-of-fit with model complexity to avoid over-fitting. In the present study, the proposed data-driven approach is employed on data from a direct numerical simulation (DNS) of a three-dimensional premixed n-heptane/air jet flame. As a result, we single out the most plausible model form of the cell reacting fraction, expressed as a function of the Damköhler number. Further, the model's ability to generalize properly to new, previously unseen data is tested. The results demonstrate the ability of the machine learning approaches to infer robust corrections for turbulence-chemistry reactor-based physics-based models.