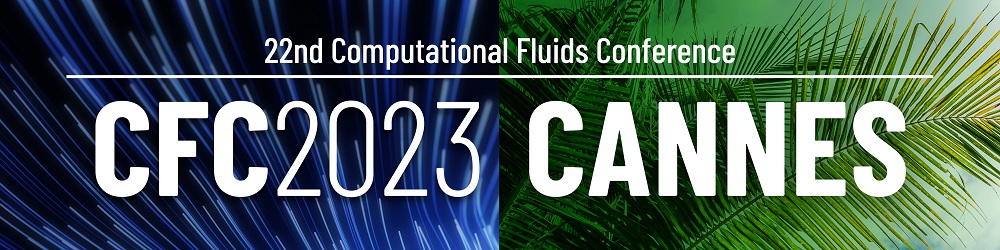
Data enhanced reduced order methods for turbulent flows
Please login to view abstract download link
The focus of the present contribution is on Reduced Order Methods (ROMs) applied to the study of turbulent flows. To ensure a low ROM computational cost, relatively few basis functions are usually considered to build the model. However, when a marginally-resolved modal regime is adopted, the number of modes is enough to capture the underlying dynamics of the system, but the standard Galerkin-ROM yields inaccurate results. In order to improve the results in terms of accuracy, the ROM approach is combined with stabilization and data-driven techniques. Specifically, we propose the first pressure-based data-driven variational multiscale ROM (P-DD-VMS-ROM), in which the available data are used to construct the so-called correction terms appearing in both the momentum and the continuity equations. Such terms are aimed at reintroducing the contribution of the neglected modes in the reduced equations. These are computed by solving an optimization problem between the exact correction term and a postulated ansatz chosen to model it. The case study considered in our numerical investigation is that of the two-dimensional flow past a circular cylinder. Two different velocity-pressure coupling approaches are used. In the first one, additional supremizer modes are added to the velocity POD space to ensure the fulfillment of the inf-sup condition. In the second approach, the continuity equation is replaced by the Pressure Poisson Equation, leading to the formulation of novel pressure correction terms. A different type of data-driven technique is combined with the VMS-ROM formulation in order to include the turbulence treatment at the reduced order level. A reduced eddy viscosity field is modelled with regression techniques starting from the velocity field making use of machine learning tools. The techniques we discuss yield significantly more accurate results than the standard ROM and, more importantly, than the original data-driven variational multiscale ROM (i.e., without pressure components). In particular, our numerical results show that adding the correction term in the momentum equation significantly improves both the velocity and the pressure approximations. On the other hand, adding the novel pressure correction terms only improves the pressure approximation.