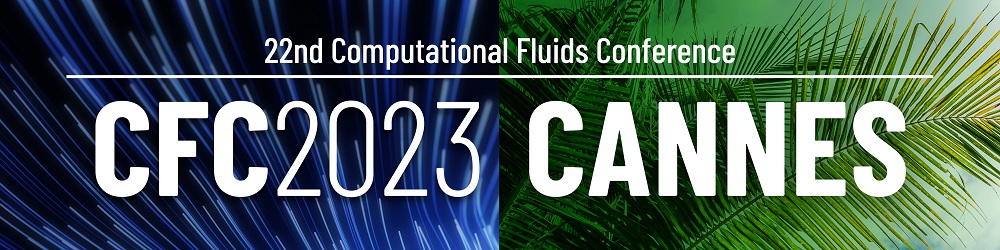
Fast data assimilation of sparse measurements for time extrapolation of main flow patterns
Please login to view abstract download link
Monitoring and control of fluid systems during operation can require on-the-fly fluid flow estimations. However, dense measurements (e.g. PIV) can hardly be continuously generated during system operation, only few sensors are generally available. To extrapolate these measurements in space, additional information -- from physics and/or previous datasets -- are often necessary. Reduced order models (ROM) are natural candidates for this task. Through data assimilation, we can couple ROM simulations with online sensors' measurements, and eventually obtain real-time 3D flow estimations. Our ROM consists in a POD-Galerkin method with a stochastic multi-scale closure [3]. The closure accurately quantifies model errors and hence strongly facilitates the data assimilation -- even far from the training set. Our method greatly exceeds the state of the art, for ROM degrees of freedom smaller than 10 and moderately turbulent 3D flows (Reynolds number up to 300) [2]. The methodology was first implemented and tested with Matlab and the finite-difference CFD code Incompact3D [1]. Recently, we have obtained similar results with the finite-volume code OpenFOAM and the ROM library ITHACA-FV [4]. We are now addressing higher Reynolds numbers and the assimilation of experimental data. REFERENCES [1] S. Laizet and E. Lamballais. High-order compact schemes for incompressible flows: A simple and efficient method with quasi-spectral accuracy. Journal of Computational Physics, 228(16):5989– 6015, 2009. [2] V. Resseguier, M. Ladvig, and D. Heitz. Real-time estimation and prediction of unsteady flows using reduced-order models coupled with few measurements. Journal of Computational Physics, 471:111631, 2022. [3] V. Resseguier, A. M. Picard, E.M´emin, and B. Chapron. Quantifying truncation-related uncertainties in unsteady fluid dynamics reduced order models. SIAM/ASA Journal on Uncertainty Quantification, 9(3):1152–1183, 2021. [4] G. Stabile and G. Rozza. Finite volume POD-Galerkin stabilised reduced order methods for the parametrised incompressible navier–stokes equations. Computers & Fluids, 173:273–284, 2018.