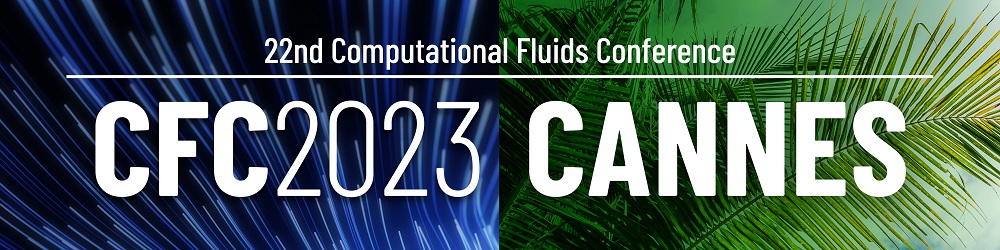
Machine Learning for Enhancing and Complementing CFD Simulations: Potentials and Limitations for Industrial Applications
Please login to view abstract download link
Machine learning and artificial intelligence techniques have transformed our everyday life within the past few years. In areas for which vast amounts of data are available the aforementioned techniques had a tremendous success, especially when mathematical models are lacking. Instead, engineering tools in general and computational fluid dynamics tools in particular rely on first-order principals that directly enable to describe and investigate system behavior. Based on these principals, derived tools significantly contribute to the green transformation of the aviation sector. However, such tools are far from perfect and suffer several short-comings, e.g. computational bottlenecks once a massive amount of simulations is required or the problem of deriving accurate turbulence models to describe small scale turbulent behavior. Machine learning techniques are generally regarded as a possibility to enhance and complement first-order based numerical simulation tools to circumvent these shortcomings. Following this ambition, the Center for Computer Applications in AeroSpace Science and Engineering department of the German Aerospace Center has investigated and industrialized scientific machine learning techniques within the past two decades always in close connection to established numerical simulation tools as well as industrial needs. This presentation will provide an insight into previous and current activities within the department covering topics from purely data-driven approaches to the incorporation of physical knowledge into models. Namely, reduced order techniques, fusion of data from different sources and with different sparsity, data-driven turbulence modeling as well as physics-informed neural networks will be discussed. Highlights will be show-cased and existing limitations outlined. Moreover, Open questions that the community has to address to further establish machine learning methods for industrial applications will be posed.