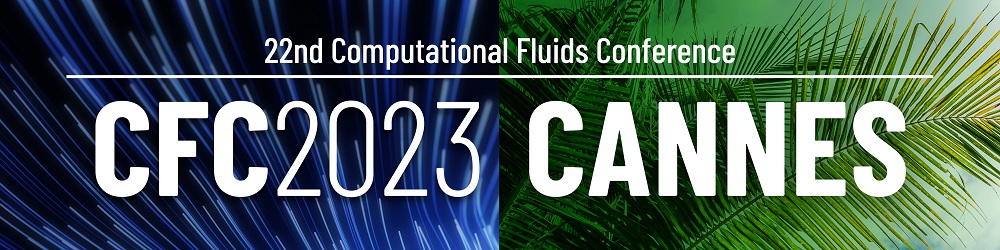
Physics-constrained Deep Learning for the k-epsilon Turbulence Model Calibration
Please login to view abstract download link
Numerical analyses of fluid flows usually rely on the Reynolds-Averaged Navier-Stokes (RANS) equations that are solved into a discretized domain. However, due to the nonlinearity of the convective term, the averaging process yields to an unclosed set of equations. The most used turbulence model to close the RANS equations is the k-epsilon where two additional transport equations are solved for the turbulent kinetic energy and the turbulent dissipation rate. As the model does not strictly rely on first principles, several empirical constants are introduced to calibrate the solution for canonical flows. The calibration of those constants is usually case-dependent and requires numerous numerical simulations. However, the computational cost of such processes can be prohibitive for many engineering applications. Deep Learning with embedded physics has shown to be a promising tool for inverse problem in fluid dynamics. In this research, we introduce a general framework for the estimation of the k-epsilon turbulence model constants via a physics-constrained deep learning architecture to leverage the computational cost of solving multiple times the governing equations into a discretized domain. The ability of the present methodology is firstly demonstrated through the estimation of the nominal constants of the k-epsilon model with RANS data as input and secondly with LES or DNS data as input. The results show that the architecture is able to predict accurately the RANS nominal coefficients with 4% of relative error and that an optimized calibration of the constants with high-fidelity data helps to improve the predictive capabilities of low-fidelity simulations for several test cases.