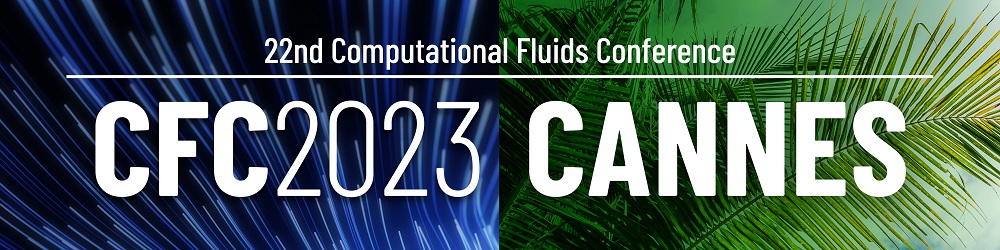
MS1-09 - Learning models for CFD: opportunities and limitations
The popularity of machine learning to compute models from data is sensitively growing in the science and engineering community. Machine learning might also offer tremendous opportunities to compute models from data for computational fluid dynamic (CFD) problems. However those opportunities come with a variety of open challenges which make the direct application of popular artificial learning methods unfeasible to obtain reliable models directly from data for applications to computational fluid problems. For instance, a first challenge is associated with the need for a large number of observations required to learn models from; a second challenge is associated with the difficulties to characterize the reliability of the predictions obtained with those models. More recently approaches have been proposed to address these challenges leveraging the synergies between scientific computing and computer science communities, and bringing the physics constraints into the learning process. Examples of these methods include, but are not limited to, computational frameworks and algorithms for multifidelity/multisource (active) learning [1], data driven model reduction methods [2], formulations for physics based machine learning, [3] and physics informed neural networks [4]. The objective of this MS is to explore the specific challenges that emerge and limit the use of data driven methods and machine learning techniques for CFD, while rising the awareness about the latest progresses in the community to overcome those limitations. The MS aims at bringing together a diverse community of researchers to discuss insights on methods and applications, and share different perspectives and ideas. The discussion of applications across a variety of engineering problems and scientific applications is welcome to encourage cross-fertilization.