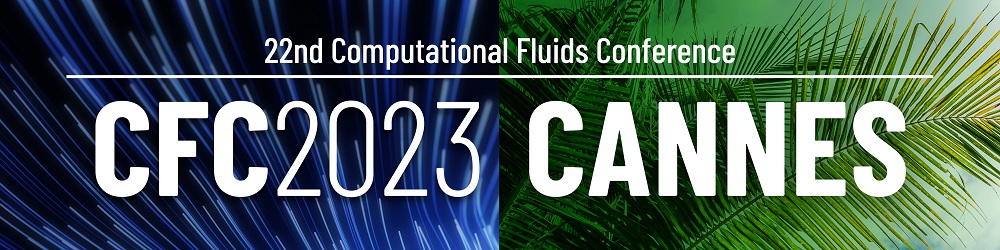
MS1-08 - Equation- and Data-driven Reduced-order Modeling for Fluid Flows
Model order reduction is a rapidly developing field of fluid dynamics that seeks to improve simulations and enhance our understanding of complex flow phenomena. Such a reduction is often unavoidable as the direct identification of bifurcations, nonlinear interactions, state transitions, non-trivial attractors in high-dimensional CFD models in computationally unfeasible, if not unattainable. Beyond their use in explaining flow phenomena in a simplified manner, reduced-order models also hold great promise in design optimization and flow control. Due to the recent advances in numerical simulations and experimental methods, data-driven model-reduction ideas are also becoming feasible in addition to equation-based techniques.
Both equation- and data-driven reduced-order modeling methods have their respective advantages and disadvantages. While machine learning and artificial intelligence offer new and powerful approaches to reduced-order modeling, they often lack physical interpretability and suffer from overfitting. On the other hand, purely equation-based modeling suffers from computational complexity, convergence issues and overly stringent assumptions.
This minisymposium seeks to present a broad view on the state of the art in model reduction methods for fluid flows, ranging from purely equation-driven to entirely data-driven approaches. Topics to be covered include long-short term memory networks [1], deep neural networks [2], as well as their physics-informed counterparts [3], Dynamic Mode Decomposition (DMD) [4] and its variants, Koopman-mode expansion [5], sparse regression methods (e.g., SINDy) [6], reduction to spectral submanifolds (SSMs) [7], and resolvent analysis [8].