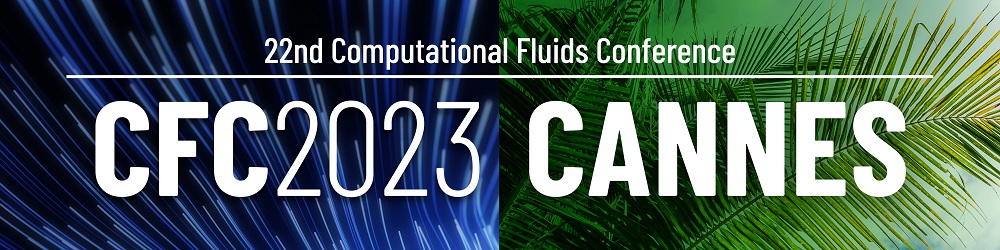
MS3-01 - Climate modeling through scientific computing and machine learning
Earth system modeling poses a wide range of computational challenges. The dynamics of many
climate components, e.g. atmosphere, ocean, land ice and sea ice, are governed by fluid flow
equations, and characterized by different spatial and temporal scales and specific physical properties
that need to be preserved by numerical models. This demands the use of a wide range of non-trivial
spatial discretizations, including mesh-based methods like spectral element, finite volume and finite
element methods, and particle-based methods such as the discrete element and material point
methods, together with advanced temporal discretizations. Moreover, these discretizations need to be
amenable to running efficiently on emerging heterogeneous architectures.
Climate models are also characterized by complex physical processes that are not fully resolved (e.g.
cloud formation) and are modeled with parameterizations. Several efforts are being made to replace
these parametrizations with data-driven models trained using observational or simulation data. More
in general, machine learning models are being used to enhance the current models with deep learning
models for improving numerical stabilization, turbulence models or for replacing parts of the climate
models with inexpensive surrogates.
In this mini-symposium we focus on computational approaches for fluid problems arising in climate
modeling, ranging from advanced discretizations and their implementation for heterogeneous
architectures, to machine learning approaches for enhancing climate models.