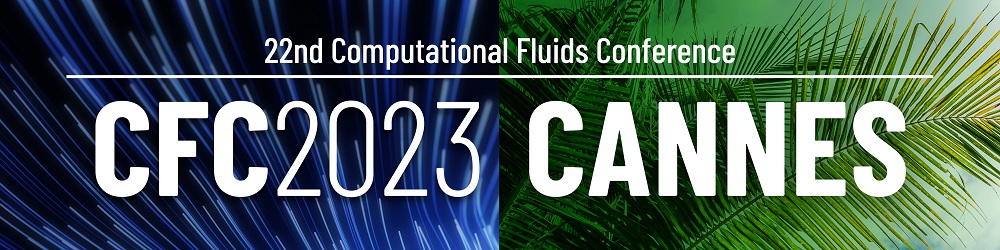
MS1-03 - Data-Driven Modeling and Machine Learning for Multiphysics Simulations
This mini-symposium focuses on the integration of computational fluid mechanics and machine learning (ML) for multiphysics simulations and coupled problems. Due to the coupled nonlinear, high-dimensional and multiscale nature, high-fidelity multiphysics simulations (e.g., fluid-structure interaction) are prohibitively expensive and challenging for parametric analysis, design optimization and control of large-scale engineering systems. On the other hand, general-purpose black-box ML techniques are computationally efficient and scalable but do not perform well beyond the data they are being trained and they lack physical interpretability for engineering applications. To address these challenges, contributions and new achievements in algorithm design and software development for hybrid physics-based ML (PBML) techniques for fluid mechanics are solicited. Critical assessment and improving conventional ML techniques to develop accurate and robust surrogate models and the integration of model order reduction (MOR) with high-fidelity multiphysics simulations and their application for real-world problems are appreciated.
This mini-symposium aims to provide a platform for investigators to disseminate and discuss PBML and data-driven MOR techniques for multiphysics prediction and design in aerospace, marine, bio-medical, geophysical flows and among others. Novel physics-based and data-driven approaches for active feedback control, real-time monitoring, multidisciplinary design optimization and uncertainty quantification, broadly related to fluid mechanics, are desired. Furthermore, new ideas for software development, implementation, and high-performance computing are welcome.