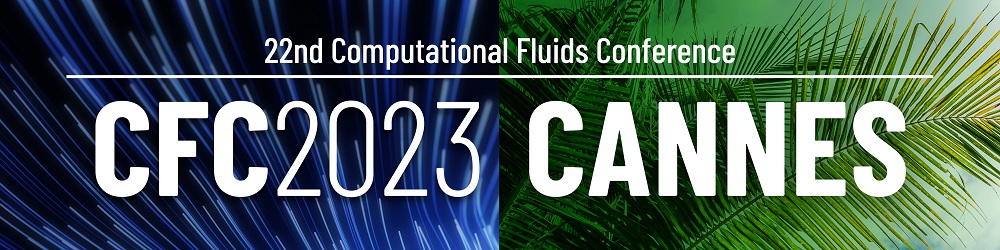
MS1-05 - Machine Learning in CFD
Due to their versatile and growing capabilities, artificial intelligence and machine learning methods have sparked great enthusiasm in most, if not all, areas of science and engineering. Concerted efforts in the CFD community are ongoing to capitalize on the phenomenal benefits of machine learning. These intense efforts, along with the availability of enormous volumes of data and large-scale simulations are reshaping the future of CFD. This mini-symposium aims to capture the strengths that machine learning can bring to CFD, highlight recent progress, prompt discussions on open questions and become a springboard for future developments. The scope is to highlight the latest developments and applications of machine learning in broad areas of CFD, including, but not limited to, algorithmic advancements (supervised, semi-supervised, unsupervised, physics-informed, etc.), exploitations (flow feature extraction, turbulence modeling, super-resolution, prediction, parsimonious nonlinear models, etc.) and applications (multi-physics simulation, large-/multi-scale simulation, control and optimization, biophysics and bioengineering, renewable energy, etc.).