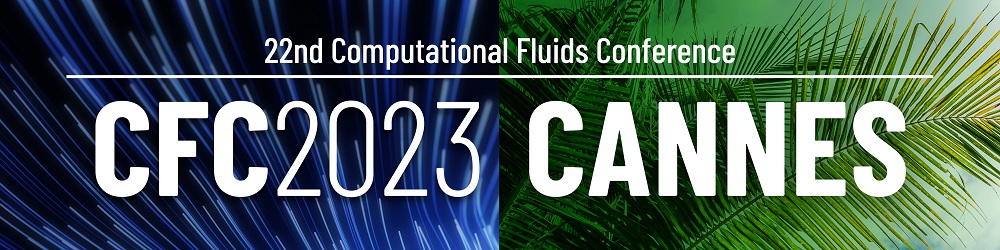
MS6-02 - Error Estimation and Adaptive Methods in CFD
Error estimation and adaptation in Computational Sciences and Engineering aim at developing efficient
methods to assess the accuracy of computer simulations and to design optimal adaptive strategies for
reliable predictions of physical phenomena. Virtually all discretization methods in PDE solvers require
the use of meshes or nodal distributions over computational domains. Defining optimal distributions
of points is therefore of paramount important for reducing computational costs when considering, for
example, multi-query simulations, such as is the case in uncertainty analysis or design optimization.
Today, the topic also extends beyond classical discretization error assessment and mesh refinement. It
also encompasses adaptive modeling with the objective of adaptively enriching surrogate models that
can arise from concurrent coupling techniques, model reduction, or response surface techniques. It
further includes novel topics relevant to engineering applications, such as goal-oriented methods, for
the control of errors in quantities of interest, or the control of errors in uncertainty analyses.
The objectives of the mini symposium will be to present the latest contributions to a posteriori error
estimation and adaptive methods with applications to computational fluid mechanics. We anticipate
contributions on the following topics:
1. Estimation of discretization and modeling errors for linear, nonlinear, or time-dependent problems,
2. Stability, convergence, and optimality analysis of adaptive methods,
3. r-adaptivity based on optimization formulations, moving mesh methods, or optimal transport,
4. Goal-oriented approaches,
5. Control of errors in Multiphysics and Multiscale problems,
6. Adaptive approaches in model reduction techniques,
7. Error estimation and adaptive schemes for uncertainty quantification,
8. Use of machine learning techniques for error estimation and adaptivity.