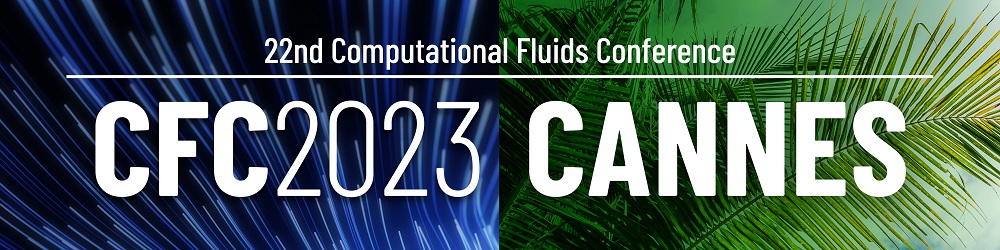
MS1-01 - Data-based technologies for modelling, informing and augmenting learning about fluids and flow simulations
Fluid mechanics, as all other branches in science and engineering is experiencing the data-revolutions. Thus, complex phenomena, encapsulated in some input-outputs pairs, can be modelled from the observed data, by using state-of-the-art and advanced machine learning technologies. In some cases, the learning process can be empowered by including existing knowledge within the process of learning: the thermodynamics consistency (energy conservation and entropy production), by including momentum and mass conservation, by including some choices of structural variables in complex fluids, … The use of these techniques allows enhancing, among many others:
• The description of complex fluids (with complex non-Newtonian rheology) including several conformational coordinates, with theirs associated evolution equation, characterizing rich nano and microstructures (suspensions, polymers, reinforce polymers, composites, …)
• Improving turbulence modeling and simulation.
• Improving computational efficiency within a Model Order Reduction setting, while ensuring accuracy and stability, for addressing large-scale simulations.
• Performing multi-scale simulation with the associated scales bridges.
• Creating parametric surrogates.
• Creating adaptive discretization techniques, empowering usual discretization techniques.
• Addressing fluid-solid interaction.
• Conciliating physics-based and data-driven models within a hybrid paradigm: digital twins, while assimilating data by using advanced technologies (e.g. neural-Kalman, …)
• Augmenting models by discovering internal (non-observed) variables and the models governing them that impact the observed dynamics.
• Taking profit of the accurate real-time responses for using that models in immersive virtual, augmented or hybrid reality.
• Novel data-driven techniques for flow control